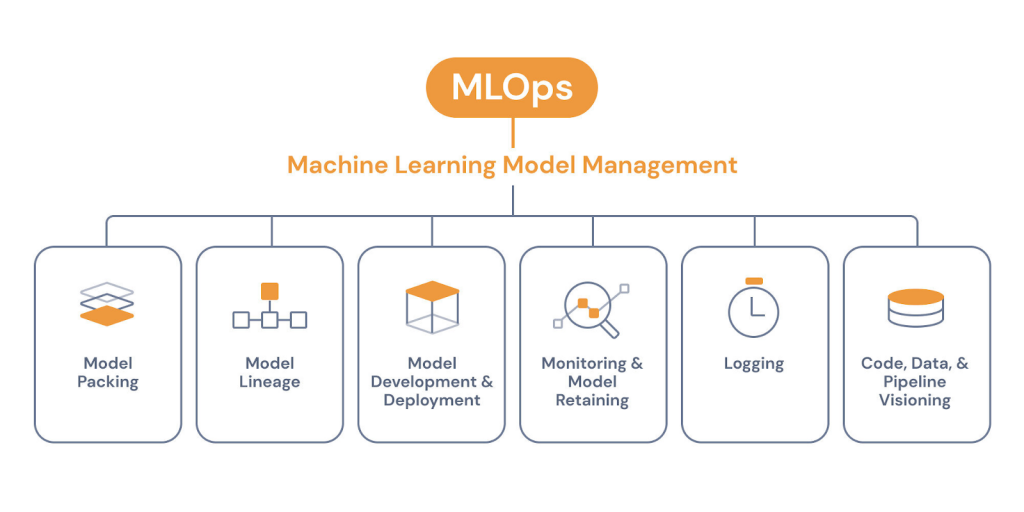
Machine learning is a complex and rapidly growing field that has the potential to revolutionize industries across the world. However, building a successful machine learning model requires much more than just writing code. It requires understanding of the data, AI algorithms, infrastructure, deployment, testing, and maintenance. This is where MLOps comes in, MLOps is a set of practices and tools that helps automate, manage, and streamline the machine learning development process.
If you want to get started with MLOps, there are various training programs available online that can teach you the basics and provide you with hands-on experience. One such program is the MLOps course, which is designed to provide students with the skills required to effectively manage and deploy machine learning models.
The MLOps course covers a wide range of topics such as data management, model building, training, testing, deployment, and monitoring. It teaches you how to use popular MLOps tools like Git, Docker, Kubernetes, and Jenkins, which are essential for managing machine learning projects. These tools help in version control, building and testing models in a consistent environment, and automating deployment pipelines.
The MLOps course is designed to be hands-on, which means that students will get plenty of opportunities to work with real-world datasets and algorithms. This not only helps students to learn better but also gives them confidence in their abilities to build and deploy machine learning models.
Overall, the mlops tutorial for beginner is an excellent investment for those who want to pursue a career in machine learning or data science. It is especially suitable for software professionals who want to transition from traditional software development to machine learning. The MLOps course provides students with the knowledge and skills required to build and deploy machine learning models in a fast and efficient manner. In today’s data-driven world, it is essential to have these skills to stay competitive.
Machine learning has been revolutionizing the world of technology, automating tedious tasks and reducing human error. But the increasing demand for machine learning models has led to challenges in deploying, monitoring, and maintaining these models over time.
To tackle these challenges, the concept of MLOps (Machine Learning Operations) has emerged as a new field that includes the use of DevOps practices to automate the building, testing, deploying, and managing of machine learning models.
MLOps is the process of applying DevOps principles to the machine learning lifecycle, making it possible to streamline the flow of data between the development and production environments. MLOps course is available to help Data Science, Operations and IT specialists learn about MLOps principles and practices.
The MLOps course teaches learners how to build a stable, scalable, and reliable machine learning system, from data preparation to model training and deployment. The course covers topics like data version control, continuous integration/continuous deployment (CI/CD) pipelines, infrastructure management, and monitoring of machine learning models in production.
MLOps course includes hands-on lab sessions that allow learners to practice setting up and configuring MLOps pipelines using popular tools like Git, Docker, Kubernetes and Jenkins. This practical training helps learners gain hands-on experience in building and deploying end-to-end machine learning pipelines.
By taking the MLOps course, learners can learn how to leverage the latest technologies to optimize the delivery and performance of machine learning models. They can also learn how to identify and address common MLOps challenges, such as model drift, data bias, security and governance.
In conclusion, MLOps is a critical aspect of modern machine learning systems, making it possible to deploy, manage and scale machine learning models in a reliable, secure, and efficient manner. By taking an MLOps course, learners can gain the knowledge, skills, and confidence to implement MLOps practices in their own organization. The course helps in meeting the growing demand for machine learning solutions and in enabling the success of machine learning models in production.